An investigation in to the effects of information provision on driver learning
- Started
- 1st October 2010
- Ended
- 31st July 2013
- Research Team
- James Snowdon
- Investigators
- Ben Waterson, Hans Fangohr
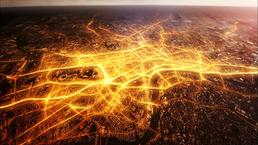
This image, showing taxi driver route choices through central London (brightness of road indicates higher usage), highlights the impact of each driver choosing their route based on information available to them from personal experience, information system
Understanding driver response to Advanced Traffic Information Systems is key to the successful design of modern transportation networks. While driver route choice and subsequent expected traffic flows have been forecast and modelled using a variety of techniques, such as aggregate utility and game theoretic analysis, we intend to demonstrate that individual vehicle-driver based models stand alone in being able to capture the complex reciprocal interactions between drivers and their environment.
Empirical studies have shown the extent of seemingly suboptimal driver behaviour, formed from imperfect network knowledge and perception. While provision of information should in theory alleviate these problems drivers will always hold some degree of apparently random behaviour (such as network exploration and exploitation of little known 'rat runs') which we intend to measure through experimental analysis.
We will utilise microscopic simulation packages, which generate spatial models consisting of heterogeneous vehicle-driver agents traversing a network, to directly generate localised delays and incidents which impact on a driver's unique experience and are learnt over time. The results of these simulations will allow us to challenge common simplifying assumptions held by modellers, particularly that transportation networks are constantly in a state of equilibrium.
Categories
Socio-technological System simulation: Social Networks, Transport
Algorithms and computational methods: Agents
Simulation software: Aimsun
Transdisciplinary tags: Complex Systems, Software Engineering